Deepfakes – essentially putting words into another person's mouth in a really believable way – have gotten more sophisticated and harder to detect each day. Current examples of deepfakes include: Taylor Swift nude picturesa Audio recording of President Joe Biden telling New Hampshire residents to not vote, and a Video of Ukrainian President Volodymyr Zelensky called on his troops to put down their arms.
Although corporations have developed detectors to detect deepfakes, studies have shown this to be the case Distortions in the information The methods used to coach these tools may end in inappropriately targeting certain populations.
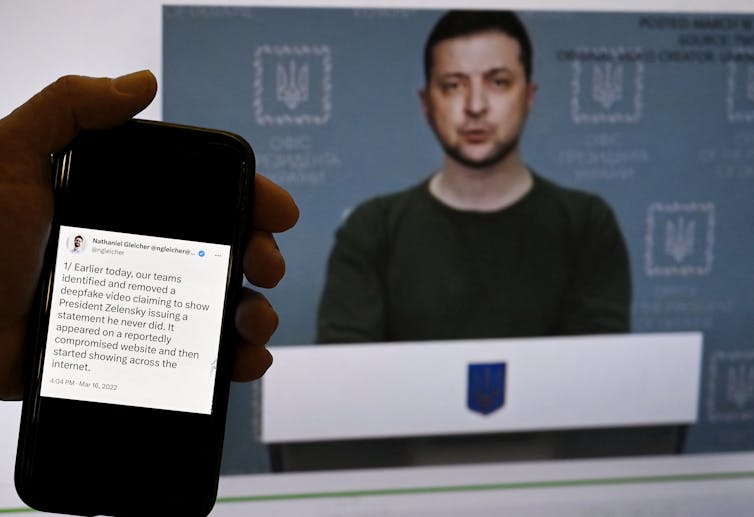
Olivier Douliery/AFP via Getty Images
My team and I even have discovered recent methods that improve each the fairness and accuracy of deepfake detection algorithms.
To do that, we used a big dataset of facial fakes, which allows researchers like us to coach our deep learning approaches. Our work is predicated on the state-of-the-art Xception detection algorithm, a widespread foundation for deepfake detection systems and might detect deepfakes with an accuracy of 91.5%.
We have developed two separate deepfake detection methods is meant to advertise justice.
One focused on making the algorithm more sensitive to demographic diversity by labeling records by gender and race to attenuate errors in underrepresented groups.
The other aimed to enhance equity without counting on demographic labels, as a substitute specializing in characteristics invisible to the human eye.
It turned out that the primary method worked best. It increased accuracy rates from 91.5% to 94.17%, which was a bigger increase than our second method in addition to several others we tested. In addition, accuracy was increased while improving fairness, which was our important focus.
We consider that fairness and accuracy are critical if the general public is to just accept artificial intelligence technology. When large language models like ChatGPT “hallucinate,” they’ll maintain erroneous information. This affects public trust and safety.
Likewise, fake images and videos can undermine AI adoption if they can not be detected quickly and accurately. An essential aspect of that is improving the fairness of those detection algorithms in order that certain demographic groups aren’t disproportionately harmed.
Our research addresses the fairness of deepfake detection algorithms, not only attempting to balance the information. It offers a brand new approach to algorithm design that takes demographic fairness into consideration as a core aspect.
image credit : theconversation.com
Leave a Reply