The human body continually creates a wide range of signals that could be measured from outside the body portable devices. This biosignal von Heart rate as much as sleep and blood oxygen mirror can indicate whether someone is Have mood fluctuations Or could be used to diagnose a wide range of Body or Brain disorders.
It could be relatively low cost to gather many organic signal data. Researchers can organize a study and ask the participants to make use of a conveyable device for a number of days with a smartwatch. However, as a way to teach an algorithm for machine learning to seek out a relationship between a certain biosignal and a health disorder, you should first teach the algorithm to acknowledge this disorder. Here computer engineers like me come into play.
Many business smartwatches, resembling One from Apple, AliveCor, Google and Samsungcurrently support the detection of atrial fibrillation. Attachment glimmer is a standard style of irregular heart rhythm, and it may well result in a stroke. One approach to recognize mechanically Atrial fibrillation should train an algorithm for machine learning to see what contores look in the info.
This approach for machine learning requires large biosignal data records during which instances of atrial fibrillation are characterised. The algorithm can use the marked authorities to learn to acknowledge a relationship between the organic signal and atrial fibrillation.
The labeling process could be quite expensive, since experts resembling cardiologists are required to undergo thousands and thousands of information points and to discover any instance of atrial fibrillation. The same problem extends to many other biosignals and disorders.
In order to resolve this problem, the researchers have developed latest opportunities to coach algorithms for machine learning with fewer labels. By first training a machine learning model to fill out the gaps of non-relevant organic signal data, the machine learning model is ready to learn the connection between a organic signal and a disturbance with fewer labels. This is known as preparation. Preparations even help mechanical learning to learn a relationship between a biosignal and a disturbance whether it is presented on a totally independent biosignal.
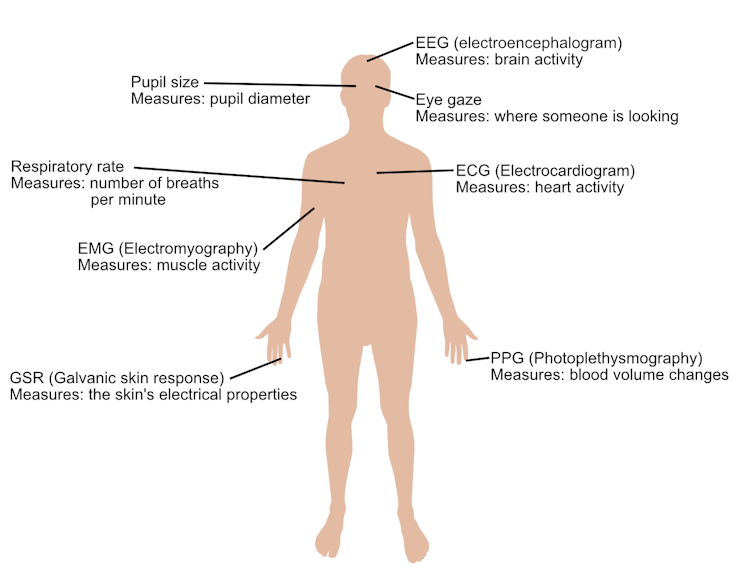
Eloy never 12 months
Challenges working with biosignals
Finding relationships between biosignals and disorders could be difficult resulting from noise or irrelevant data, a distinction between the biosignals of individuals and since the connection between a biosignal and a disorder will not be clear.
First, biosignals contain a variety of noise. For example, when you wear a smartwatch while running, the watch moves. This implies that the sensor records at different points in the course of the run at various points. Since the locations vary in the middle of the run, the fluctuations within the organic signal value can now be attributed to fluctuations within the recording location as an alternative of physiological processes.
Second, the biosignals of all are unique. The location of the veins, for instance, often differs between people. This implies that the organic signal connected to those veins, even when smartwatches are worn at the identical place in any respect wrists. The same underlying signal as the guts rate of an individual results in different organic signal values.
The underlying signal itself can be unique for people or groups of individuals. The resting heart rate of a mean person is around 60-80 strokes per minute, but athletes can have a resting frequency Up to 30-40 strokes per minute.
After all, the connection between an organic signal and a disturbance is commonly complex. This implies that the disorder isn’t immediately apparent whenever you take a look at the organic signal.
Algorithms for machine learning enable researchers to learn from data and to bear in mind the complexity, noise and variability of individuals. By using large biosignal data records, machine learning algorithms can find clear relationships that apply to everyone.
Learn to fill out the gaps
Researchers can use unrolled organic signal data as warming up for the algorithm for machine learning. This warm up, or PrerequisitePrepared the algorithm for machine learning to seek out a relationship between the biosignal and a disorder. This is a bit like walking around a park to get the landing before you train a path to run.
There are some ways to arrange an algorithm for machine learning. In My research With Dolby Laboratories researcher Lies LU And Previous researchThe algorithm for machine learning is taught fill out the gaps.
To do that, we take a biosignal and artificially create gaps with a certain length-for example a second. We then teach the algorithm for machine learning to fill out the missing piece of organic signal. This is feasible since the algorithm for machine learning sees what the organic signal looks like before and after the gap.
If an individual's heart rate in front of the gap is about 60 strokes per minute, there’ll probably be a heartbeat within the gap of 1 second. In this case, we train the algorithm for machine learning to predict when this heartbeat occurs.
As soon as we have now trained the algorithm for machine learning, it would have found a relationship between the guts rate of an individual and the following time. We can now train the algorithm for machine learning with this relationship between a traditional heart rate and an already learned biosignal. This makes it easier for the algorithm to learn the connection between heart rate and atrial fibrillation. Since atrial fibrillation is characterised by fast and irregular heartbeats and the algorithm can now predict well if a heartbeat occurs, it may well quickly learn to acknowledge these irregularities.
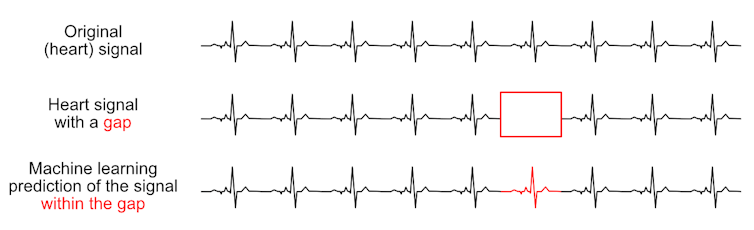
Eloy never 12 months
The idea of filling out the gaps can be generalized to other biosignals. Previous research has shownAnd our work confirmed that the preliminary model of a model with out a organic signal without labels can learn clinically useful relationships from other organic signals with just a number of labels. This link implies that researchers in biosignals which can be easy to gather the machine learning model for many who are difficult to gather are easy to gather.
Recognition development faster
By improving the preparation, researchers could make mechanical learning algorithms higher and more efficiently to discover diseases and disorders. Improvements before the priority reduce the prices and the time spent by expert labels.
A current example of algorithms for machine learning for early detection is Google's Pulse loss Smartwatch function. The aspiring area of biosignal pre -image may also help to enable a faster development of comparable features with a wider area of biosignals and a wider series of disorders.
With increasing forms of biosignals and more data, researchers may discover relationships that dramatically improve the early detection of diseases and disorders. The earlier diseases and disorders are found, the higher a treatment plan works for patients.
image credit : theconversation.com
Leave a Reply