Blood samples from patients infected with a parasitic worm that causes schistosomiasis contain hidden information that characterizes different stages of the disease. In ours recently published research resultsOur team used machine learning to uncover this hidden information and improve early detection and diagnosis of infections.
The parasite that causes schistosomiasis completes its life cycle in two hosts – first in snails after which in mammals equivalent to humans, dogs and mice. Freshwater worm eggs enter the human host through the skin and flow into throughout the body. Damage to multiple organsincluding liver, intestines, bladder and urethra. When these larvae reach the blood vessels that connect the intestines to the liver, they mature into adult worms. They then release eggs, that are excreted throughout the infected person's bowel movements. Continuation of the transmission cycle.
Current since diagnosis is predicated on the detection of eggs in fecesDoctors often miss the early stages of infection. By the time eggs are discovered, the disease is already at a complicated stage. Because diagnosis rates are poor, public health officials typically turn to diagnosis Mass administration of the drug praziquantel for the population within the affected regions. However, praziquantel cannot eliminate young worms in early stages of infection and forestall reinfection.
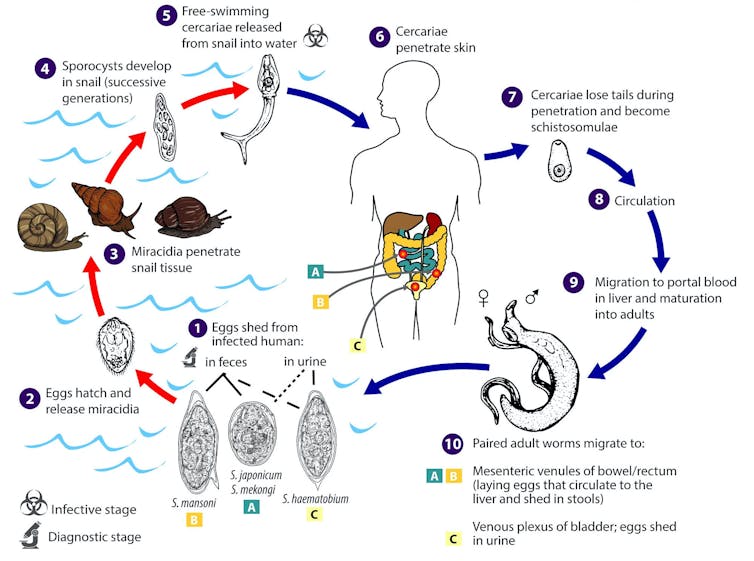
DPDx/CDC
Our study provides a transparent path to improving early detection and diagnosis Identifying the hidden information within the blood This signals an energetic infection within the early stages.
Your body responds to a schistosomiasis infection by mounting an immune response involving multiple varieties of immune cells in addition to antibodies that specifically goal molecules secreted or present by the worm and eggs. Our study presents two ways to screen for specific features of antibodies that indicate early infection.
The first is an assay that detects a quantitative and qualitative profile of the immune responseThese include different classes of antibodies and properties that determine how they impart with other immune cells. This allowed us to discover specific facets of the immune response that distinguish uninfected patients from those with early and late stages of the disease.
Second, we developed a brand new one Machine learning approach that analyzes antibodies to discover latent features of the immune response which are related to disease stage and disease severity. We trained the model on immune profile data from infected and uninfected patients and tested the model on data not used for training and on data from a unique geographic location. We have identified not only biomarkers for the disease, but in addition the potential mechanism underlying the infection.
Why it matters
Schistosomiasis is a neglected tropical disease It affects over 200 million people worldwide and 280,000 people die from it yearly. Early diagnosis can improve the effectiveness of treatment and forestall serious illness.
Furthermore, unlike many machine learning methods, that are black boxes, our approach can be black boxes interpretable. This means it will possibly provide insights into why and the way the disease develops that transcend simply identifying disease markers and may guide future strategies for early diagnosis and treatment.
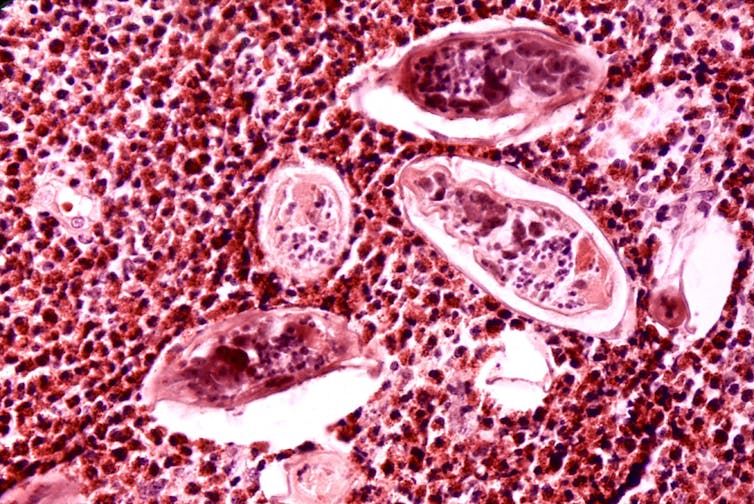
CDC/Dr. Edwin P. Ewing Jr.
What shouldn’t be yet known
The schistosomiasis infection signatures we identified remain stable in two geographic regions on two continents. Future research could examine how applicable these biomarkers are to additional populations.
Furthermore, our work identifies a possible mechanism behind disease progression. We found that a selected immune response against a selected protein on the surface of the worm signals an intermediate stage of infection. Understanding how the immune system responds to this little-understood antigen could improve diagnosis and treatment.
What's next?
Our results not only improve our understanding of how the immune system responds to different stages of infection, but in addition discover key antigens that would pave the way in which for the event of cost-effective and efficient diagnostic and treatment approaches. Our next steps include actually implementing these strategies into practice for early detection and treatment of disease.
image credit : theconversation.com
Leave a Reply